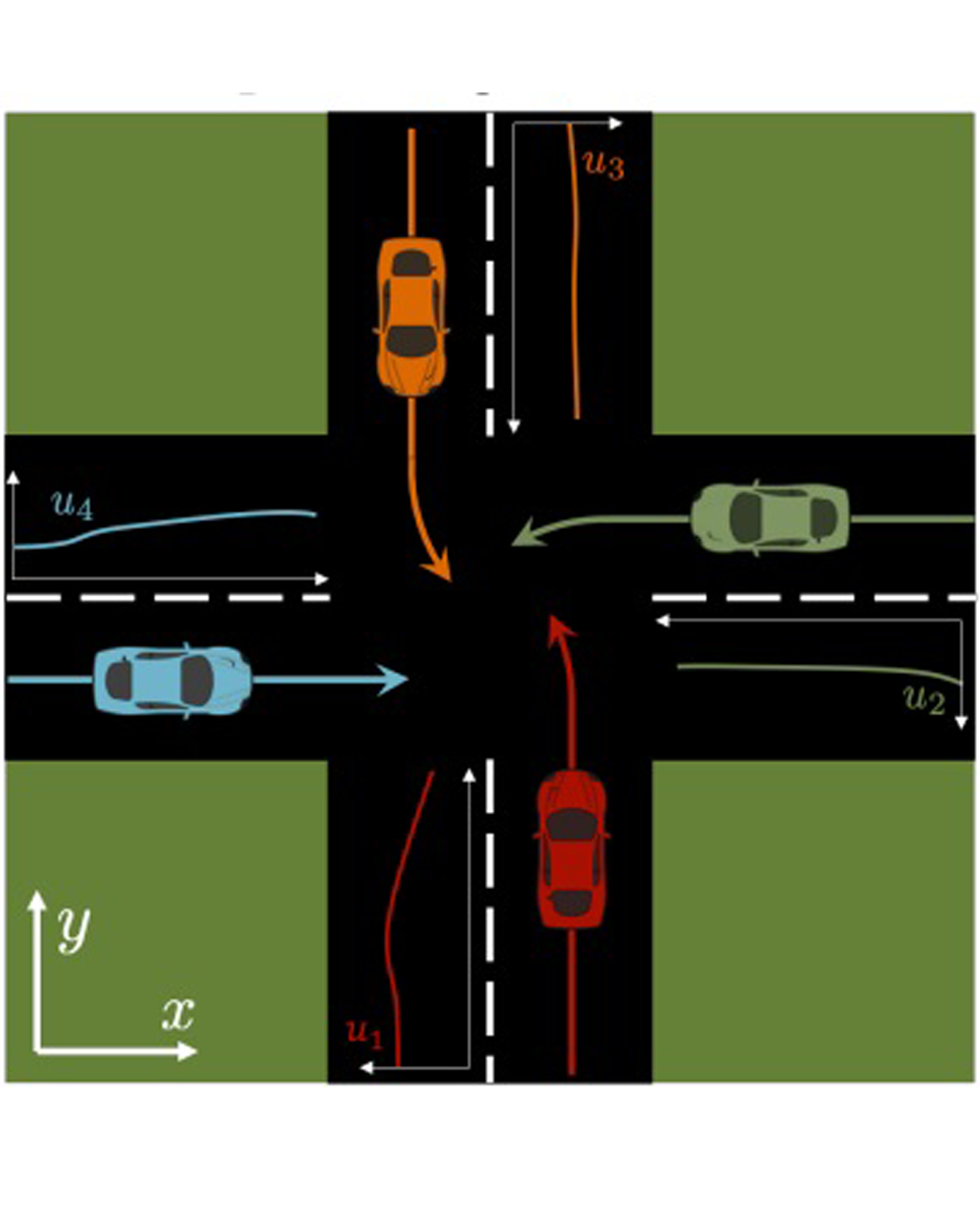
We focus on decentralized navigation among multiple noncommunicating agents at uncontrolled street intersections. Avoiding collisions under such settings demands nuanced implicit coordination. This is challenging to accomplish; the high dimensionality of the space of possible behavior and the lack of explicit communication among agents complicate prediction and planning. However, the structure of these domains often collapses the space of possible collective behavior into a finite set of modes. Our key insight is that enabling agents to reason about modes may enable them to coordinate implicitly via intent signals encoded in their actions. In this paper, we represent modes as low-dimensional multiagent motion primitives in a compact and interpretable fashion using the formalism of topological braids. Based on this representation, we derive a probabilistic model that maps past behavior of multiple agents to a future mode. Using this model, we design a decentralized control algorithm that treats navigation as uncertainty minimization over the space of modes. This algorithm enables agents to collectively reject unsafe intersection crossing strategies in a distributed fashion. We demonstrate our approach in a simulated four-way uncontrolled intersection. Our model is shown to reduce the frequency of collisions by over 65% against baselines explicitly reasoning in the space of trajectories, while maintaining comparable time efficiency in challenging scenarios. READ MORE