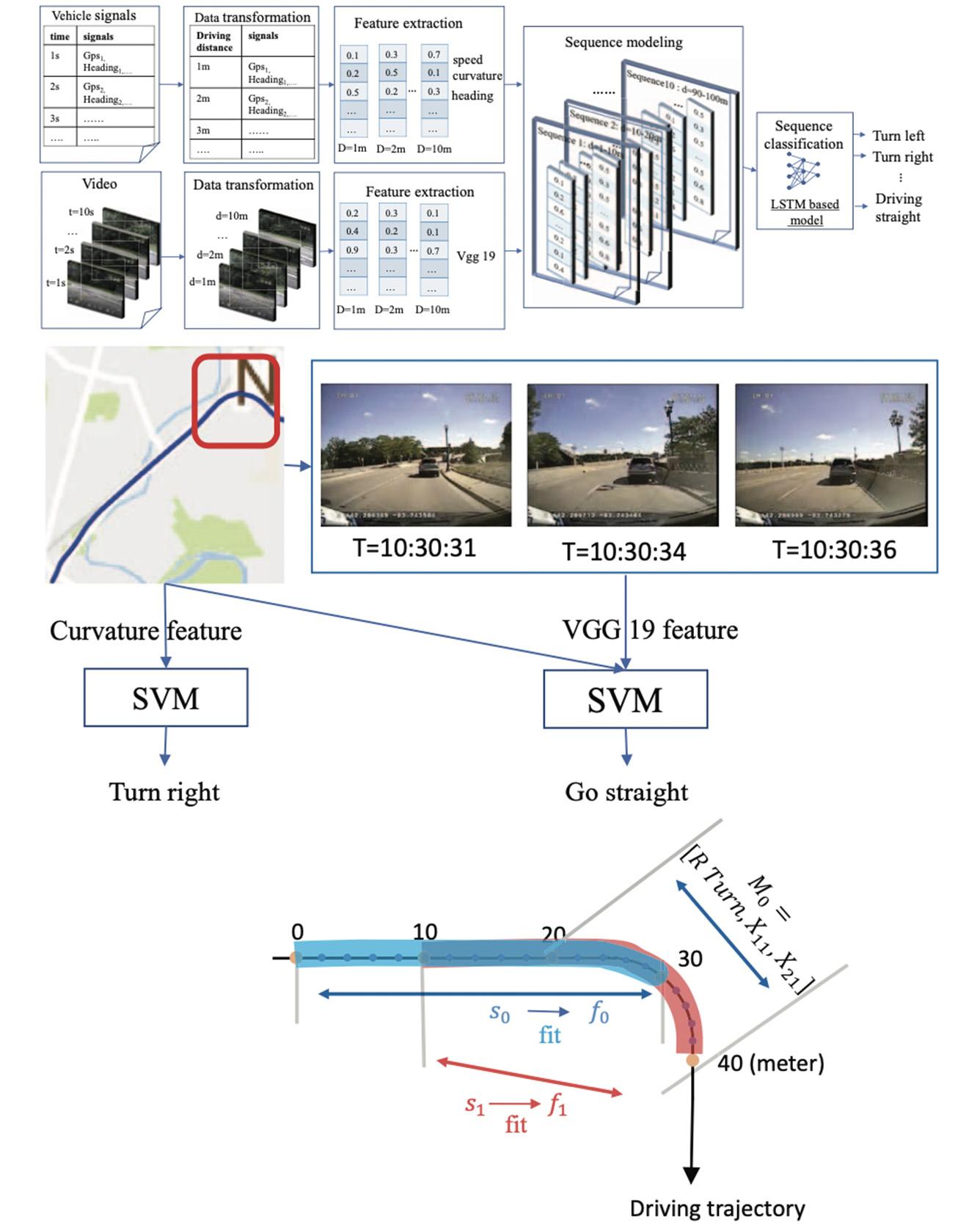
TRI Author: Simon Stent
All Authors: Xishuai Peng, Ruirui Liu, Yi Lu Murphey, Simon Stent, Yuanxiang Li
Driving maneuver detection is one of the most challenging tasks in Advanced Driver Assistance Systems (ADAS). Research has shown that the early notification of improper driving maneuvers is helpful to avoid fatalities and serious accidents. In this paper, we introduce a driver maneuvering detection (DMD) system. The DMD system contains three major computational components, distance based representation of driving context, combined features of vehicle trajectory and VGG-19 network features extracted from the video images of vehicle front view, and a Long Short-Term Memory (LSTM)-based neural network model to learn sequence knowledge in driving maneuvering events. We show through experiments that the DMD system is capable of learning the latent features of five different classes of driving maneuvers and achieving significantly better performance than traditional classification methods on real-world driving trips. Read More
Citation: Peng, Xishuai, Ruirui Liu, Yi Lu Murphey, Simon Stent, and Yuanxiang Li. "Driving maneuver detection via sequence learning from vehicle signals and video images." In 2018 24th International Conference on Pattern Recognition (ICPR), pp. 1265-1270. IEEE, 2018.