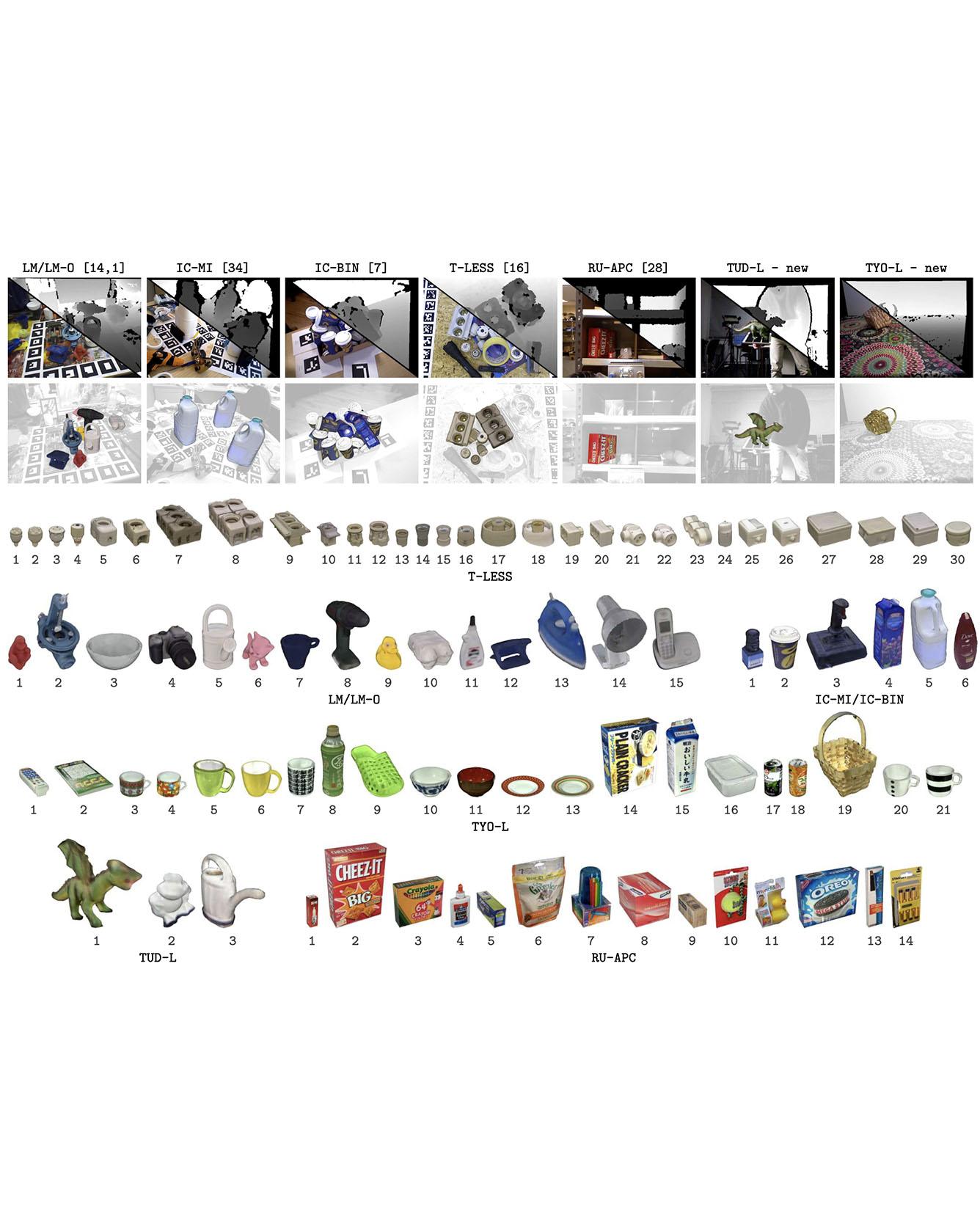
TRI Author: Wadim Kehl All Authors:Tomas Hodan, Frank Michel, Eric Brachmann, Wadim Kehl, Anders Buch, Dirk Kraft, Bertram Drost, Joel Vidal, Stephan Ihrke, Xenophon Zabulis, Caner Sahin, Fabian Manhardt, Federico Tombari, Tae-Kyun Kim, Jiri Matas, Carsten Rother
We propose a benchmark for 6D pose estimation of a rigid object from a single RGB-D input image. The training data consists of a texture-mapped 3D object model or images of the object in known 6D poses. The benchmark comprises of: i) eight datasets in a unified format that cover different practical scenarios, including two new datasets focusing on varying lighting conditions, ii) an evaluation methodology with a pose-error function that deals with pose ambiguities, iii) a comprehensive evaluation of 15 diverse recent methods that captures the status quo of the field, and iv) an online evaluation system that is open for continuous submission of new results. The evaluation shows that methods based on point-pair features currently perform best, outperforming template matching methods, learning-based methods and methods based on 3D local features. The project website is available at bop.felk.cvut.cz. Read MoreCitation: Hodan, Tomas, Frank Michel, Eric Brachmann, Wadim Kehl, Anders GlentBuch, Dirk Kraft, Bertram Drost et al. "Bop: Benchmark for 6d object pose estimation." In Proceedings of the European Conference on Computer Vision (ECCV), pp. 19-34. 2018.