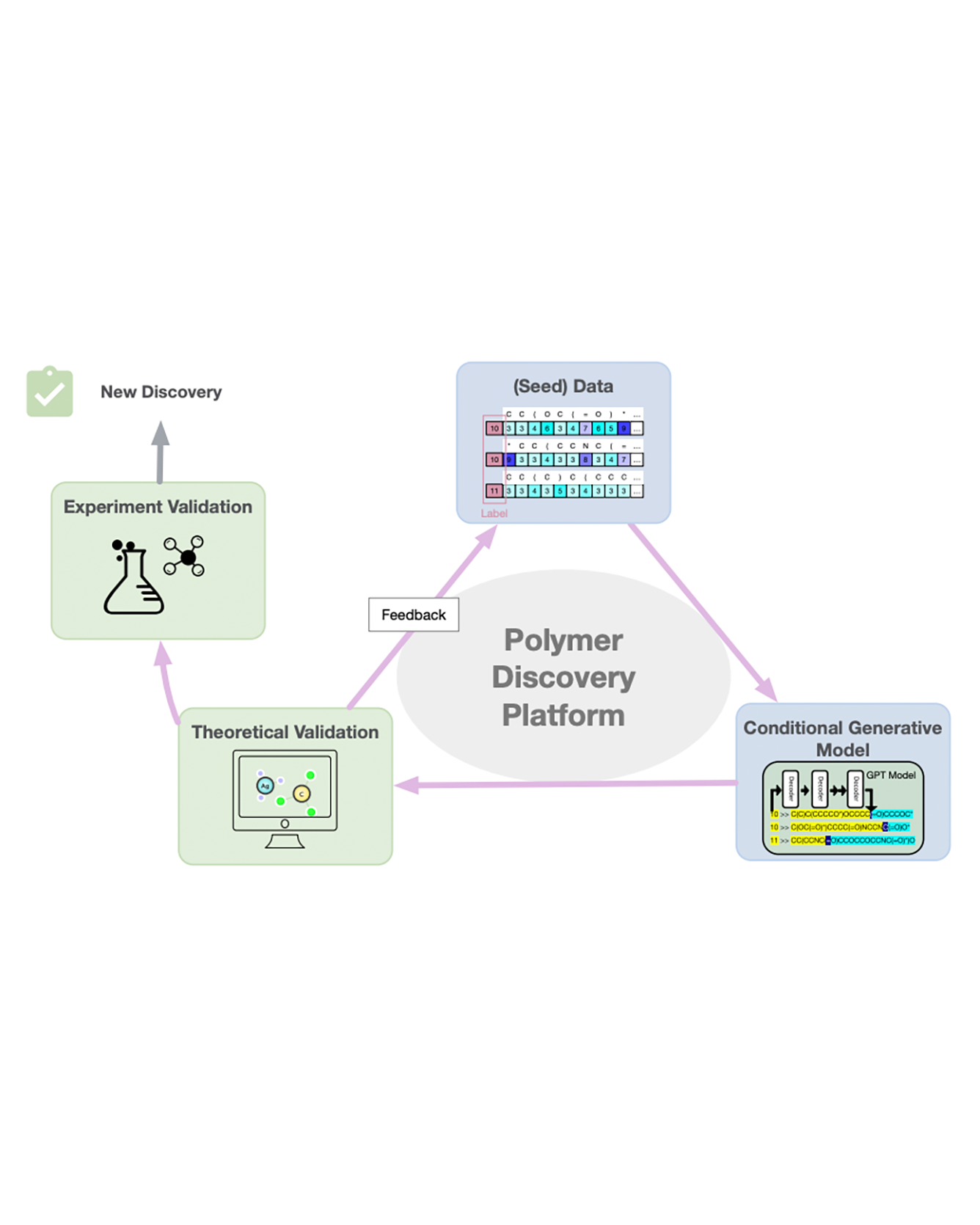
In this work, we introduce a polymer discovery platform designed to identify polymers with tailored properties efficiently, exemplified through the discovery of high-performance polymer electrolytes. The platform integrates three core components: a conditioned generative model, validation modules, and a feedback mechanism, creating a self-improving system for material innovation. To demonstrate the efficacy of this platform, it is used to identify polymer electrolyte materials with high ionic conductivity. A simple conditional generative model, based on the minGPT architecture, can effectively generate candidate polymers that exhibit a mean ionic conductivity that is significantly greater than those in the original training set. This approach, coupled with molecular dynamics simulations for validation and a specifically designed acquisition mechanism, allows the platform to refine its output iteratively. Notably, after the first iteration, we observed an increase in both the mean and the lower bound of the ionic conductivity of the new polymer candidates. The platform's effectiveness is underscored by the identification of 19 polymer repeating units, each displaying a computed ionic conductivity surpassing that of Polyethylene Oxide (PEO). The discovery of these polymers validates the platform's efficacy in identifying potential polymer materials. Acknowledging current limitations, future work will focus on enhancing modeling techniques, validation processes, and acquisition strategies, aiming for broader applicability in polymer science and machine learning. READ MORE