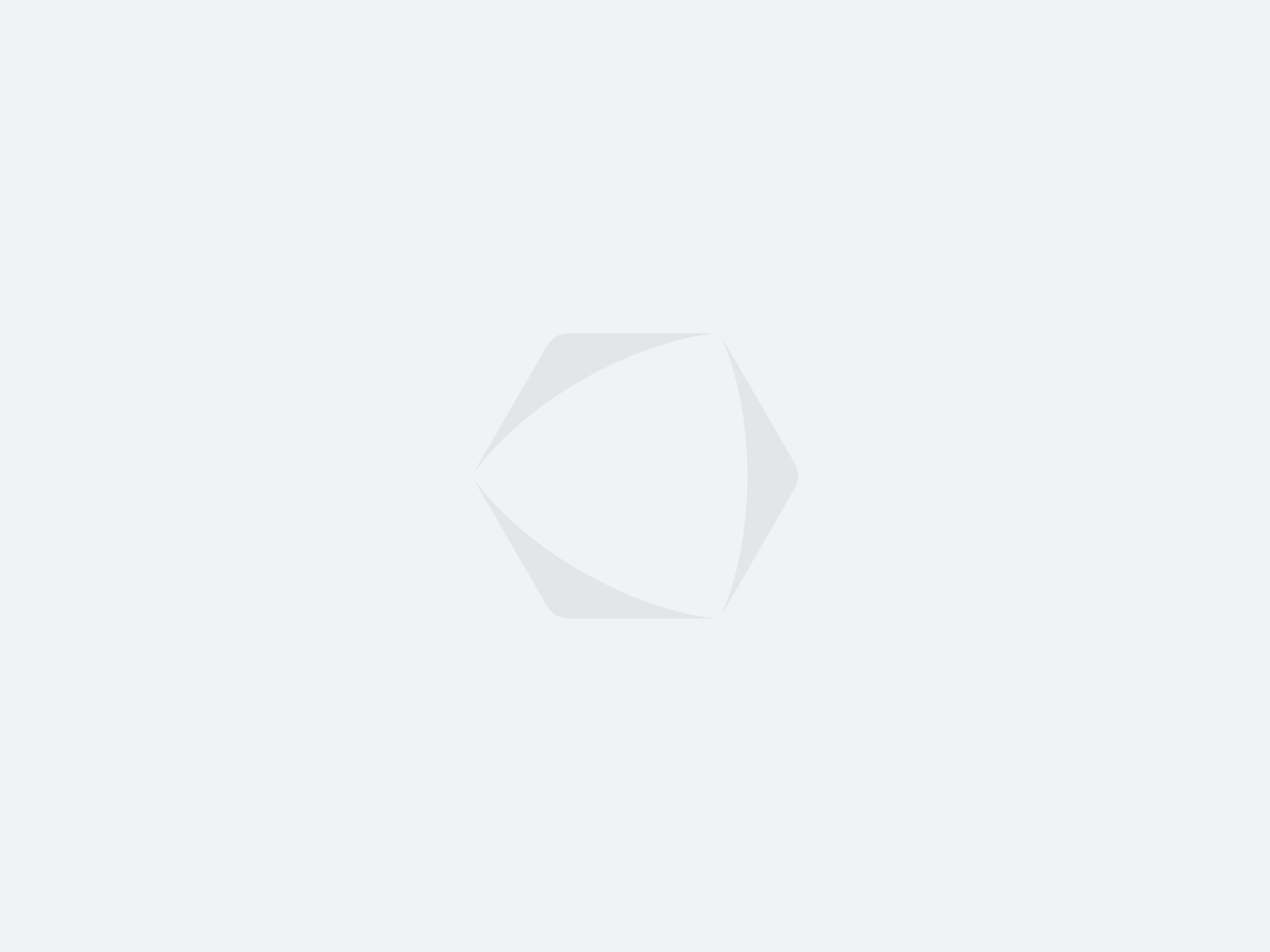
TRI Authors: Paul Ozog, Ryan W. Wolcott, Jeffrey M. Walls
All Authors: Fernando Nobre, Christoffer Heckman, Paul Ozog, Ryan W Wolcott, Jeffrey M Walls
Sparse feature-based maps provide a compact representation of the environment that admit efficient algorithms, for example simultaneous localization and mapping. These representations typically assume a static world and therefore contain static map features. However, since the world contains dynamic elements, determining when map features no longer correspond to the environment is essential for long-term utility. This work develops a feature-based model of the environment which evolves over time through feature persistence. Moreover, we augment the state-of-the-art sparse mapping model with a correlative structure that captures spatio-temporal properties, e.g. that nearby features frequently have similar persistence. We show that such relationships, typically addressed through an ad hoc formalism focusing only on feature repeatability, are crucial to evaluate through a probabilistically principled approach. The joint posterior over feature persistence can be computed efficiently and used to improve online data association decisions for localization. The proposed algorithms are validated in numerical simulation and using publicly available data sets. Read More
Citation: Nobre, Fernando, Christoffer Heckman, Paul Ozog, Ryan W. Wolcott, and Jeffrey M. Walls. "Online probabilistic change detection in feature-based maps." In 2018 IEEE International Conference on Robotics and Automation (ICRA), pp. 1-9. IEEE, 2018.