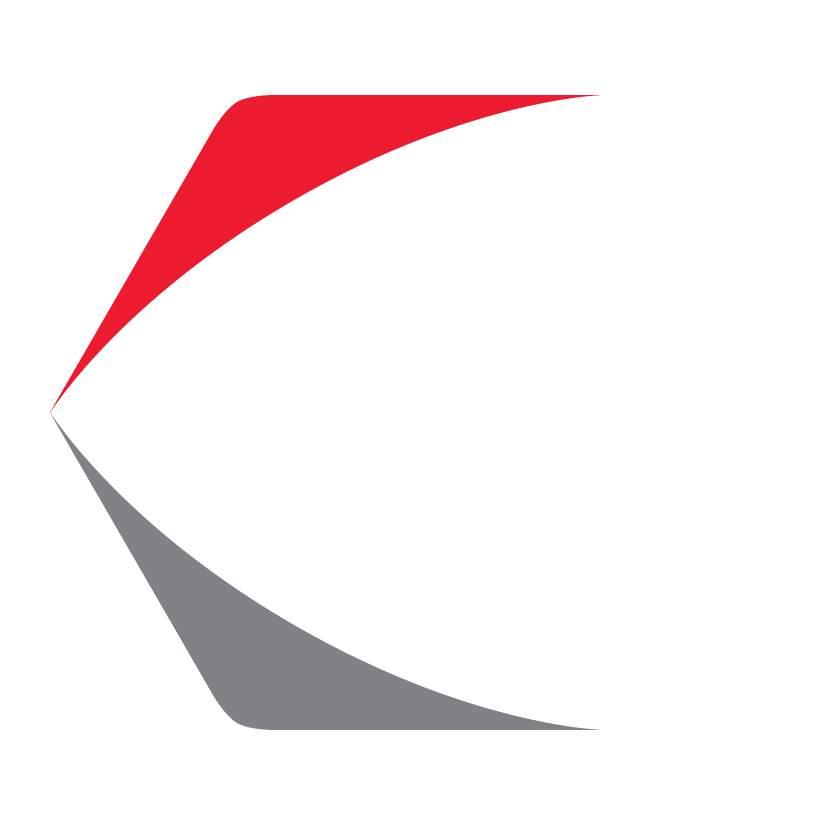
Autonomous vehicle controllers capable of drifting can improve safety in dynamic emergency situations. However, drifting involves operating at high sideslip angles, which is a fundamentally unstable operating regime that typically requires an accurate vehicle model for reliable operation; such models may not be available after environmental or vehicle parameter changes. Towards that goal, this work presents a Nonlinear Model Predictive Control approach which is capable of initiating and controlling a drift in a production vehicle even when changes in vehicle parameters degrade the original model. A neural network model of the vehicle dynamics is used inside the optimization routine and updated with online learning techniques, giving a higher fidelity and more adaptable model. Experimental validation on a full size, nearly unmodified Lexus LC500 demonstrates the increased modeling fidelity, adaptability, and utility of the presented controller framework. As the LC500 is a difficult car to drift, previous approaches which rely on physics based vehicle models could not complete the autonomous drift tests on this vehicle. Furthermore, the tires on the experimental vehicle are then switched, changing the vehicle parameters, and the capability of the controller to adapt online is demonstrated. READ MORE