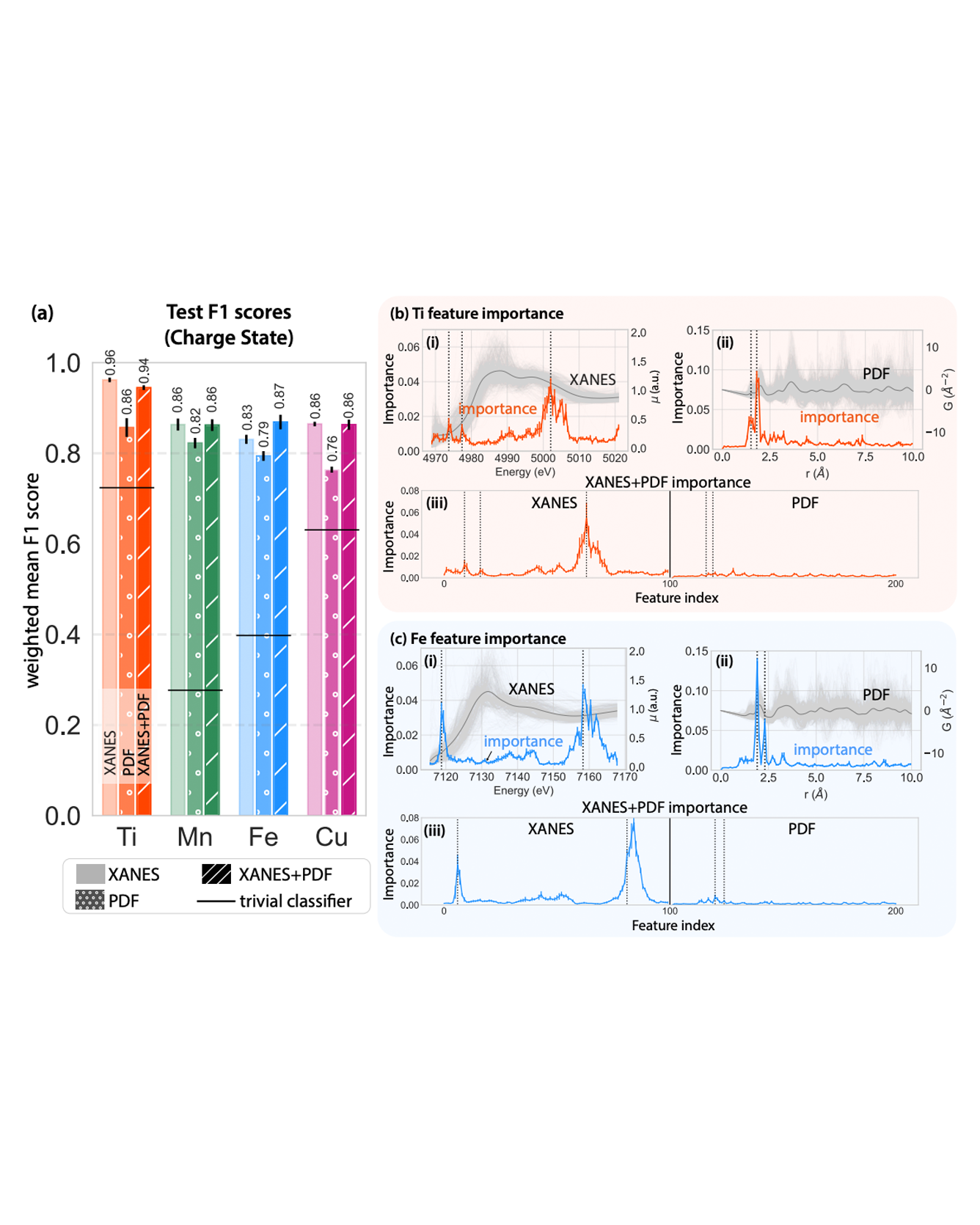
We used off-the-shelf interpretable ML techniques to combine information from multiple heterogeneous spectra: X-ray absorption near-edge spectra (XANES) and atomic pair distribution functions (PDFs), to extract information about local structure and chemistry of transition metal oxides. This approach enabled us to analyze the relative contributions of the different spectra to different prediction tasks. Specifically, we trained random forest models on XANES, PDF, and both of them combined, to extract charge (oxidation) state, coordination number, and mean nearest-neighbor bond length of transition metal cations in oxides. We find that XANES-only models tend to outperform the PDF-only models for all the tasks, and information from XANES often dominated when the two inputs were combined. This was even true for structural tasks where we might expect PDF to dominate. However, the performance gap closes when we used species-specific differential PDFs (dPDFs) as the inputs instead of total PDFs. Our results highlight that XANES contains rich structural information and may be further developed as a structural probe. Our interpretable, multimodal approach is quick and easy to implement when suitable structural and spectroscopic databases are available. This approach provides valuable insights into the relative strengths of different modalities for a practical scientific goal, guiding researchers in their experiment design tasks such as deciding when it is useful to combine complementary techniques in a scientific investigation. READ MORE