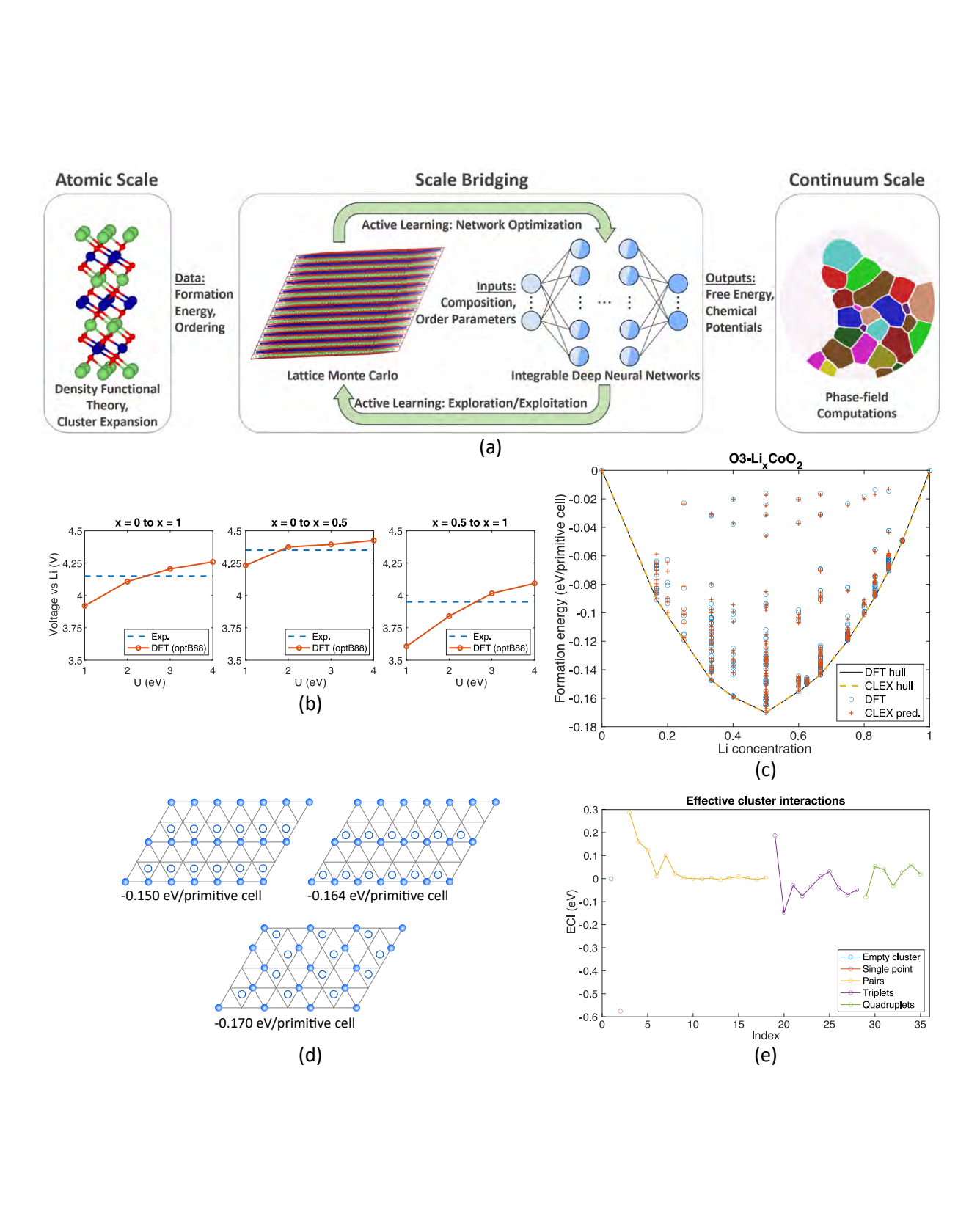
LixTMO2 (TM=Ni, Co, Mn) forms an important family of cathode materials for Li-ion batteries, whose performance is strongly governed by Li composition-dependent crystal structure and phase stability. Here, we use LixCoO2 (LCO) as a model system to benchmark a machine learning-enabled framework for bridging scales in materials physics. We focus on two scales: (a) assemblies of thousands of atoms described by density functional theory-informed statistical mechanics, and (b) continuum phase field models to study the dynamics of order-disorder transitions in LCO. Central to the scale bridging is the rigorous, quantitatively accurate, representation of the free energy density and chemical potentials of this material system by coarsegraining formation energies for specific atomic configurations. We develop active learning workflows to train recently developed integrable deep neural networks for such high-dimensional free energy density and chemical potential functions. The resulting, first principles-informed, machine learning-enabled, phase-field computations allow us to study LCO cathodes' phase evolution in terms of temperature, morphology, charge cycling and particle size. READ MORE