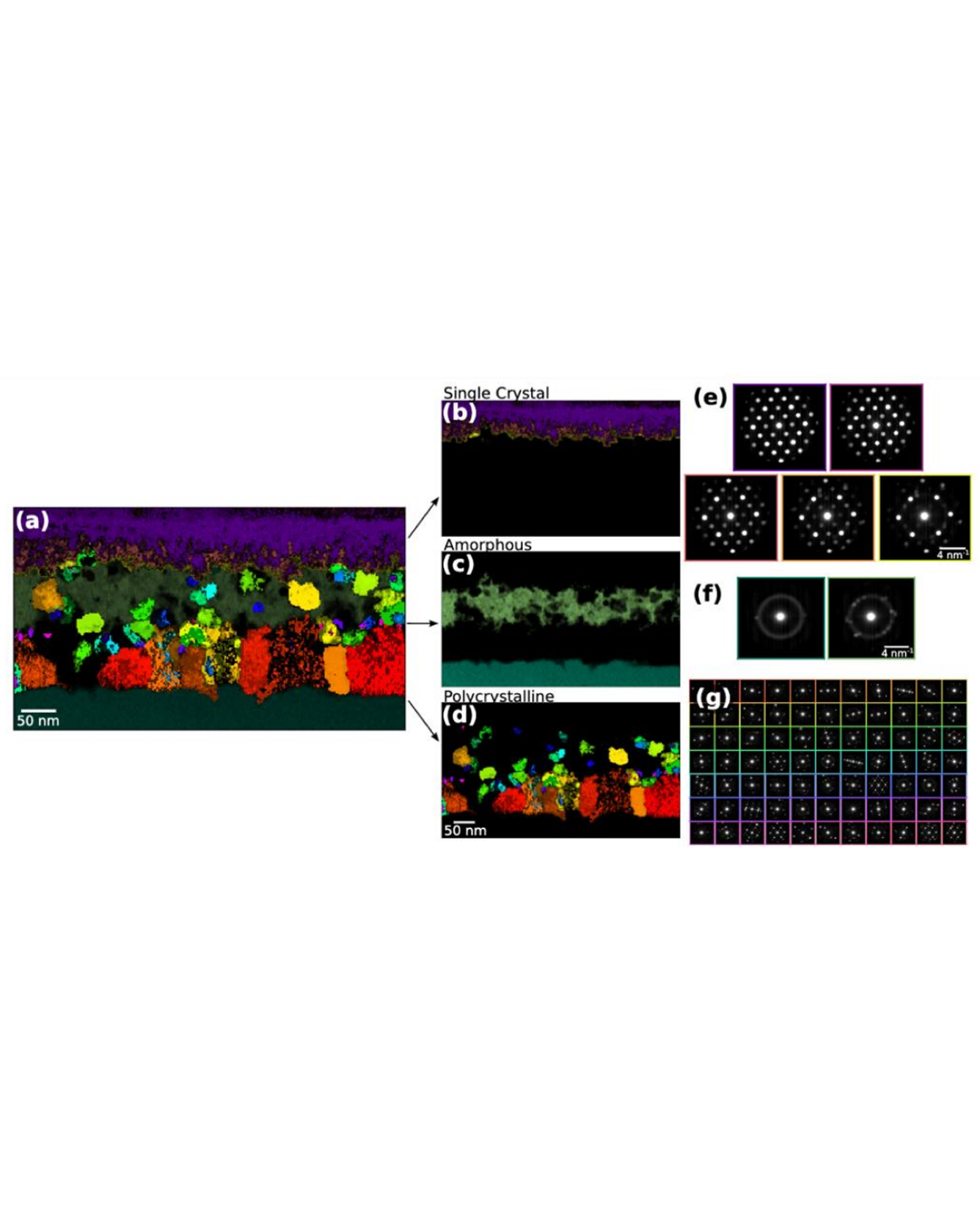
In four-dimensional scanning transmission electron microscopy (4D-STEM), a diffraction pattern is collected at each position of the electron beam in a 2D raster over the sample surface. Samples with many regions of differing order, such as samples with mixed regions of crystalline material in different orientations and amorphous regions, generate complex datasets. This data requires classification, or identification of the distinct regions, so that each region can be appropriately analyzed. Ideally, each class corresponds to a type of diffraction pattern, or to structurally meaningful features or motifs, such that a scan position will be included in a given class if and only if its diffraction pattern contains these features. Recent progress towards effective, efficient, and open source 4D-STEM classification algorithms has been rapid [1-5]. As 4D-STEM datasets commonly reach 100s of GB in size, it is necessary to judiciously reduce the data to a compressed representation before performing classification. Here, we define a feature vector calculated from measured Bragg disk positions, demonstrate its effectiveness at classifying complex 4D-STEM dataset, and discuss its potential for fully automating 4D-STEM classification. READ MORE