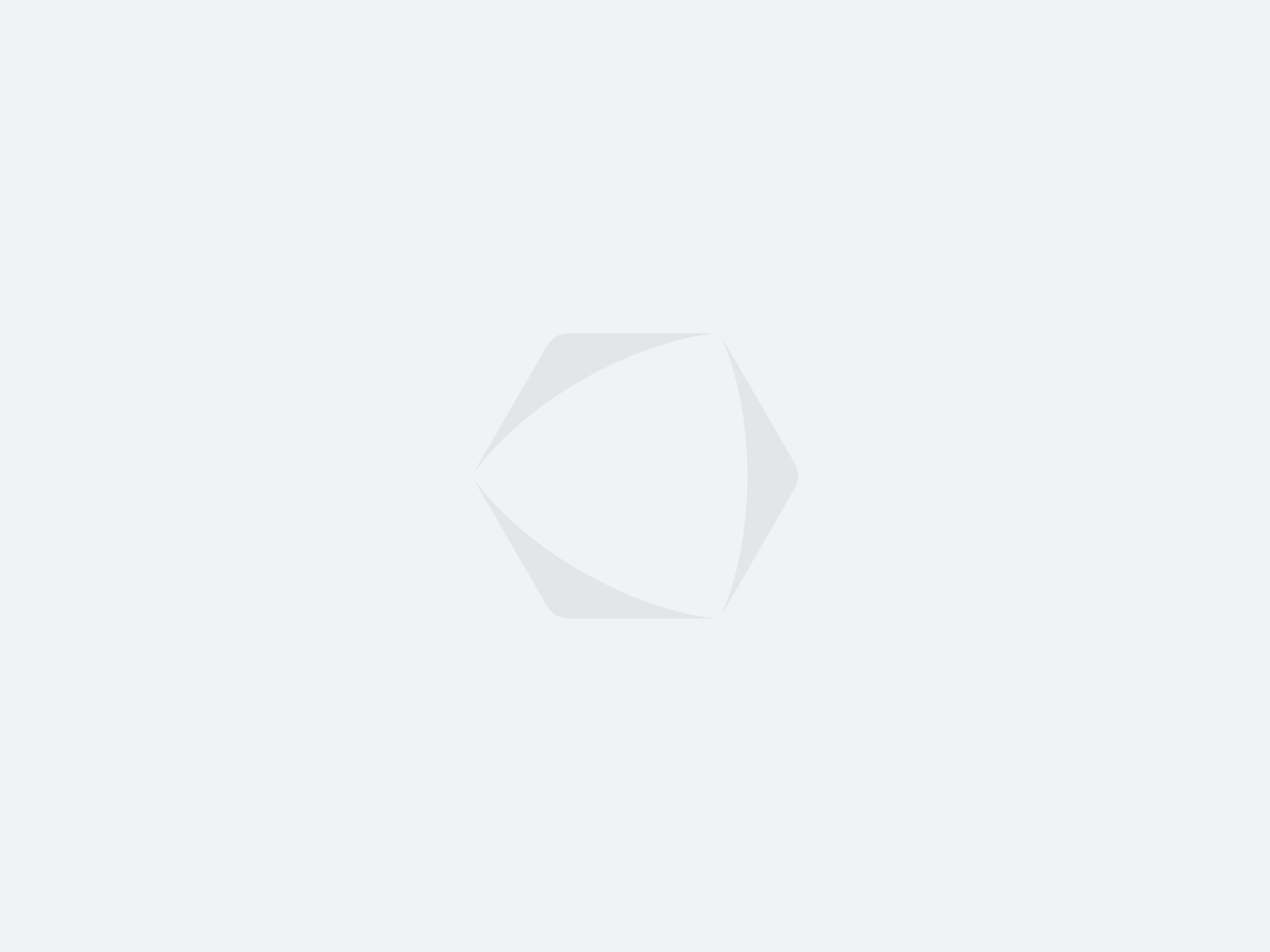
TRI Author: Adrien Gaidon
All Authors: Adrien Gaidon, Antonio Lopez, Florent Perronnin
The recent successes in many visual recognition tasks, such as image classification, object detection, and semantic segmentation can be attributed in large part to three factors: (i) advances in end-to-end trainable deep learning models (LeCun 2015), (ii) the progress of computing hardware, and (iii) the introduction of increasingly larger labeled datasets such as PASCAL VOC (Everingham et al. 2010), KITTI (Geiger et al. 2012), ImageNet (Russakovsky et al. 2015), MS-COCO (Lin et al. 2014), and Cityscapes (Cordts et al. 2016), among others. In fact, recent results (Sun et al. 2017; Hestness et al. 2017) indicate that the reliability of current visual models might not be limited by the algorithms themselves but by the type and amount of supervised data available. Therefore, to tackle more challenging tasks, such as video scene understanding, progress is needed not only on the algorithmic and hardware fronts but also on the data front, both for learning and quantitative evaluation. However, acquiring and densely labeling a large visual dataset with ground truth information (e.g. semantic labels, depth, optical flow) for each new problem is not a scalable alternative. Read More
Citation: Gaidon, Adrien, Antonio Lopez, and Florent Perronnin. "The reasonable effectiveness of synthetic visual data." International Journal of Computer Vision 126, no. 9 (2018): 899-901.